Overview
The insurance industry faces many risks. It is prone to fraud, which can lead to big financial losses, inefficiencies, and unhappy customers. Fraudulent claims hurt insurers. These claims can be exaggerated damages or made-up incidents. They make it hard for insurers to stay profitable and serve honest policyholders fairly. Fraud detection in insurance has traditionally depended on manual processes and rule-based systems. Fraud tactics are getting smarter. So, artificial intelligence (AI) is now a key tool in fighting fraud and reducing financial losses.
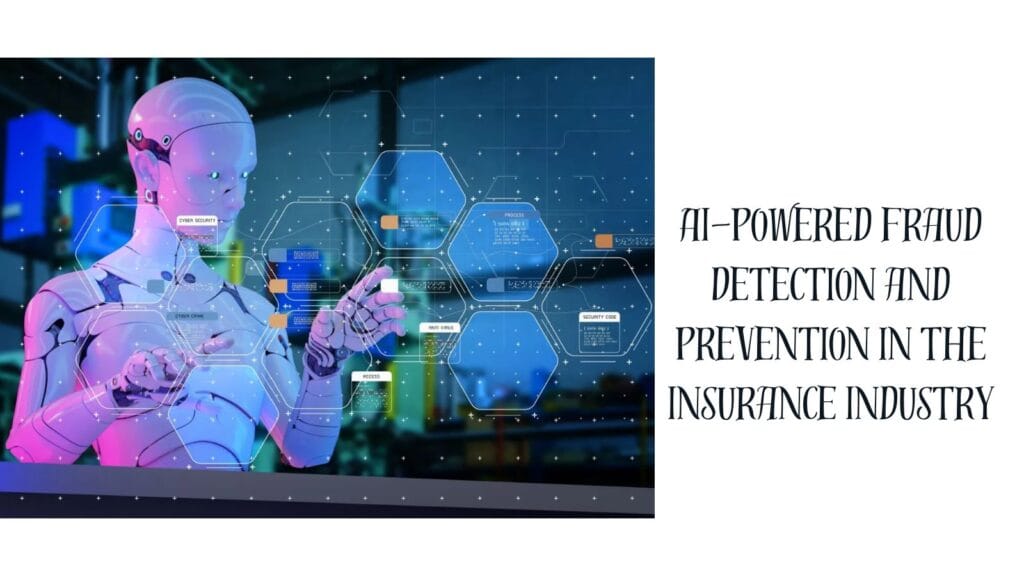
AI can handle large amounts of data, spot patterns, and adjust to new situations. This helps insurers find suspicious activities quicker and more accurately than old methods. Machine learning (ML), natural language processing (NLP), and other AI tools are changing how insurers detect fraud. These technologies help find fake claims, cut costs, and improve operations. They also boost customer experiences.
In this article, we’ll delve into the role of AI in fraud detection and prevention in the insurance industry. We’ll look at how machine learning helps find fraud. We’ll see how NLP analyzes claims documents. Then, we’ll explore real-world case studies. Finally, we’ll discuss the future of AI in cutting false claims and improving operations.
Machine Learning Algorithms for Fraud Detection
How Machine Learning Enhances Fraud Detection
Machine learning (ML) algorithms learn from data. They find patterns on their own, without needing specific programming for every situation. In fraud detection, ML models learn from past claims. This includes real cases and fraudulent ones. ML algorithms can analyze data to find patterns of suspicious activity. This helps flag potentially fraudulent claims for further investigation.
Key Types of Machine Learning Algorithms Used in Fraud Detection:
- Supervised Learning: This method trains the algorithm using labeled data. It includes examples of both fraudulent and legitimate claims. This helps the model learn to tell the difference between them. Once the model is trained, it can predict whether new, unlabeled claims are legitimate or potentially fraudulent.
- Unsupervised Learning: Here, the algorithm gets data without labels. It finds anomalies by spotting patterns in the data. This approach is particularly useful when historical data on fraud cases is limited.
- Reinforcement Learning: This ML algorithm learns by trying things out. It improves its method using feedback. Reinforcement learning boosts fraud detection accuracy by interacting with real-world data.
How Machine Learning Detects Fraud:
- Anomaly Detection: ML models can find claims that don’t fit typical patterns. This includes unusual claim amounts, unexpected timing, or odd policyholder behaviors. By identifying anomalies early, insurers can flag potentially fraudulent claims for further review.
- Risk Scoring: ML algorithms can give risk scores to claims. They consider several factors, like the claimant’s history, the type of claim, and where the incident happened. A high-risk score would indicate that the claim requires additional investigation.
Real-World Example: Shift Technology’s AI Platform
Shift Technology is an AI fraud detection company. They created a platform that uses machine learning. This platform finds fraudulent claims in various insurance types, like health, auto, and property. Shift Technology’s system analyzes past claims data. It learns to spot suspicious patterns. A key success is detecting fraud in real-time. This cuts the insurer’s risk and boosts claims management efficiency. In 2020, Shift helped an insurance client reduce fraud by 25% and improved overall claims processing times.
Natural Language Processing (NLP) for Analyzing Claims Documentation
How NLP Helps in Fraud Detection
Natural Language Processing (NLP) is a branch of AI that focuses on the interaction between computers and human language. NLP can help detect fraud in insurance by analyzing claims documents. This includes police reports, medical records, and witness statements. By examining this text, NLP can find inconsistencies and signs of fraud.
Key NLP Applications in Fraud Detection:
- Textual Analysis: NLP algorithms can check written claims documents. They find discrepancies or odd language patterns. For example, certain phrases or wording may suggest that a claim is fabricated or exaggerated.
- Sentiment Analysis: NLP can check the feelings behind a claimant’s words. It helps see if those feelings match usual emotional reactions to an event. For example, if someone says they had a traumatic car accident but seems too calm or uses strange language, NLP can mark this as suspicious.
- Document Matching: NLP can compare the contents of various documents, such as medical records, police reports, and witness statements. This helps make sure they all match. Discrepancies between documents might point to fraudulent activity.
Real-World Example: Tractable’s NLP-Driven Claims Automation
Tractable, an AI-powered startup, uses NLP to help insurers automate claims processing, particularly for auto insurance. Their system scans and analyzes photos, reports, and textual claims documents to detect fraud. Tractable uses NLP to analyze claim documents. This helps find inconsistencies that could mean a claim is false. The platform’s AI helps insurers speed up claims review. This means quicker and more accurate fraud detection. It also cuts down on the costs of checking claims manually.
Case Studies of AI Successfully Preventing Fraud in the Insurance Sector
1. AXA and the Use of AI for Fraud Prevention
AXA, one of the world’s leading insurance companies, has integrated AI into its fraud detection and prevention strategies. AXA used machine learning algorithms to analyze big data. This helped them spot claims that were different from usual patterns. This allowed AXA to flag high-risk claims for further investigation. In a pilot program, AXA cut fraudulent claims by 30%. They did this by automating detection and spotting suspicious patterns early.
AXA has also partnered with a tech firm to use AI for real-time fraud detection, making it easier to prevent fraud before it affects customers. This system has cut down on fake claims. It also makes the claims process easier for real policyholders.
2. Cigna’s AI Fraud Prevention Tools
Cigna, a global health services provider, uses AI tools to spot fraud in medical claims. Cigna uses machine learning and NLP to find strange billing patterns. This helps them catch fraudulent claims that might slip through the cracks.
Cigna’s AI tools do more than detect fraud. They also boost the efficiency of the claims process. This means real claims get processed quickly. At the same time, they help reduce losses from fraud.
The Future of AI in Reducing False Claims and Operational Costs
AI and the Evolution of Fraud Detection
As AI continues to advance, its role in the insurance industry will expand. Future developments in machine learning and NLP will allow insurers to detect fraud with even greater accuracy and speed. For example:
- Predictive Analytics: AI will get better at predicting. Insurers can spot possible fraud before it occurs. AI can spot high-risk individuals and claims early by looking at patterns and historical data.
- Self-Learning Algorithms: Machine learning algorithms will keep evolving. They will detect new types of fraud that we couldn’t find before. These self-learning algorithms adapt to new fraud tactics. They help insurers stay ahead of fraudsters.
- Blockchain Integration: Combining AI with blockchain technology could further enhance fraud detection. Blockchain’s secure ledger stops data tampering. AI finds oddities in claims data. Together, they create strong protection against fraud.
Reducing Operational Costs
AI can automate fraud detection and claims management. This reduces operational costs significantly. AI cuts down on manual work in fraud detection. This saves resources that would be used for checking suspicious claims. Faster detection means fewer payouts for fraud. This helps insurers save money overall.
The Challenge of Balancing Accuracy and Privacy
AI has great potential for fraud detection, but insurers face challenges. A big challenge is keeping data private while using personal info to detect fraud. Insurers must balance fraud prevention and protecting customer data. This is crucial due to growing concerns about data privacy laws around the world.
Conclusion
AI-powered fraud detection is changing the insurance industry. It helps insurers fight fraudulent claims better and faster than ever. Machine learning, natural language processing, and real-time data analytics help insurers. They spot suspicious claims, speed up claims processing, and cut operational costs.
As technology evolves, AI will get better at detecting fraud. This will provide more protection for both insurers and policyholders. AI integration needs careful thought about data privacy and security. Insurers must tackle these issues to fully use AI’s power in fighting fraud.
The future of AI in insurance looks bright. It aims to create a more efficient, cost-effective, and fraud-resistant industry. This change will lead to better outcomes for insurers and their customers.
Insurance in the Age of IoT (Internet of Things)
FAQs
Machine learning helps by analyzing historical claims data to identify patterns of fraud. It uses algorithms to find unusual patterns in new claims. This helps insurers quickly and accurately identify possible fraud.
NLP is a form of AI that analyzes and interprets human language. In insurance, NLP analyzes claims documents. It spots inconsistencies or suspicious language that might show fraud.
While AI greatly enhances fraud detection, it cannot entirely eliminate fraud. It greatly lowers the chance of fraudulent claims being missed. This happens by automating detection and boosting accuracy.
AI lowers operational costs by automating fraud detection. This lets insurers concentrate on real claims. Additionally, faster detection reduces payouts for fraudulent claims, leading to overall cost savings.
Future plans involve:
Predictive analytics
Self-learning algorithms
AI integration with blockchain technology
These advancements will boost fraud detection. They will make it more efficient and
Insurance companies are using AI for fraud detection. This change brings a new era of efficiency and reliability. Analyzing large data sets, spotting fraud patterns, and speeding up claims will get better. This will make insurance fraud detection smarter and more accurate.